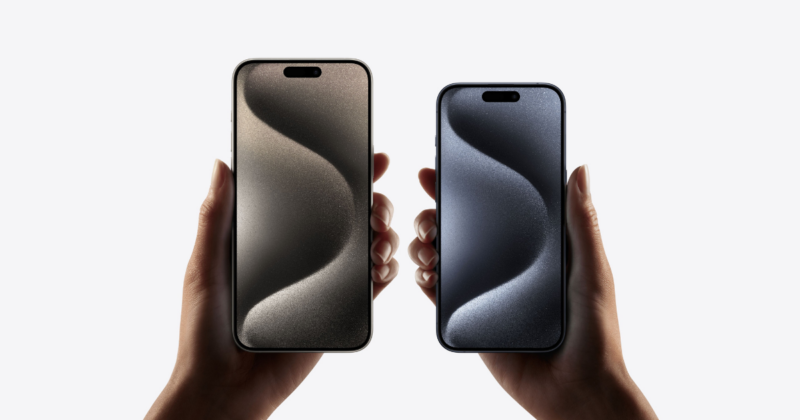
Apple could leap ahead of competitors in device AI-integration with the infusion of a new proposed animated 3D avatar and AI language learning model tech into resource-constrained devices. These could very well be the future iPhones and iPads. The Cupertino-based tech giant recently published two new papers regarding the same technologies.
Let’s take a look at what is going on in Apple engineers and tech experts in mind with the proposed technolgies.
Using HUGS to Create More-Accurate Animated 3D Models
In the first research paper, Apple has proposed a new technology called HUGS to create animated 3D avatars. HUGS stands for Human Gaussian Splats and uses a technique called 3D Gaussian Splatting, which is used to render real-time 3D models from real-life data into a 3D space.
Apple’s emphasis will be to make the tech learn to “disentangle” a static scene captured in a “monocular video with a small number of (50-100) frames” into a “fully-animatable human avatar within 30 minutes.”
Apple says in the abstract of the research paper that it will use the SMPL body model to initialize the human Gaussians. The SMPL technology cannot model sharper details like cloth and hair and that is where the 3D Gaussians come in. The tech giant says that it plans to utilize HUGS so that the 3D Gaussians “deviate from the human body model”, capturing details like hair and cloth and render animated 3D models. This approach is expected to result in more realistic and accurate-looking animated 3D avatars.
“We propose to jointly optimize the linear blend skinning weights to coordinate the movements of individual Gaussians during animation. Our approach enables novel-pose synthesis of human and novel view synthesis of both the human and the scene. We achieve
state-of-the-art rendering quality with a rendering speed of 60 FPS while being ∼100× faster to train over previous work.”, said Apple in the abstract of the research paper.
Reducing Data Load and Enhancing Memory-Usage Efficiency of Resource-Constrained Devices
In the second research paper, Apple has outlined the challenge of running large language models (LLMs) like GPT-3, OPT, and PaLM on low-memory devices. The tech giant aims to make low-memory devices more capable for advanced AI language models.
It says that since LLMs “can contain hundreds of billions or even trillions of parameters”, it is
challenging to load and run them on “resource-constrained devices.” The entire model has to be loaded into DRAM for inference which “severely limits the maximum model size that can be run.” You would need a bigger DRAM capacity to just load the model, which is not possible with resource-constrained devices.
To remedy this, Apple proposes “to store the model parameters on flash memory, which is
at least an order of magnitude larger than DRAM. Then, during inference, we directly and cleverly load the required parameters from the flash memory, avoiding the need to fit the entire model in DRAM.”
The tech behemoth proposes the use of two techniques–Windowing and Row-Column Bundling— to reduce data load on devices and also to make their memory usage more efficient.
Apple claims in the research paper that it has “demonstrated the ability to run LLMs up to twice the size of available DRAM, achieving an acceleration in inference speed by 4-5x compared to traditional loading methods in CPU, and 20-25x in GPU.”
The tech giant believes it is a crucial breakthrough in “deploying advanced LLMs in resource-limited environments, thereby expanding their applicability and accessibility.”
-
Utkarsh found his calling to be a writer while studying engineering and has been writing professionally for over six years now. After completing his graduation from MIT, Manipal, he cut his teeth in the ever-evolving media industry, working… read more